This Mind-Blowing AI Can See, Hear, AND Understand — Here’s How Multimodal AI Is Changing Everything!
Multimodal AI Explained: The Tech Behind the Hype
What Is Multimodal AI?
Multimodal AI is a branch of artificial intelligence that processes and integrates multiple types of data—such as text, images, audio, video, and sensor data—simultaneously. Unlike traditional AI models that focus on a single data type, multimodal AI combines diverse inputs to achieve a richer, more comprehensive understanding of the world, similar to how humans use multiple senses to interpret their environment.

Example of an image input for multimodal AI models like GPT-4 Vision.
Why Multimodal AI Matters
- Human-like Understanding: By fusing different data types, multimodal AI mimics how people naturally combine sight, sound, language, and other senses to interpret context and make decisions.
- Enhanced Accuracy: Integrating multiple modalities leads to more precise predictions and robust outputs compared to unimodal systems.
- Broader Applications: From healthcare to entertainment, multimodal AI unlocks new possibilities in automation, personalization, and decision-making across industries.
How Does Multimodal AI Work?
Core Components
- Data Inputs: Multimodal AI ingests various data types—text, images, audio, video, and sensor data.
- Architecture: Neural networks and deep learning models are specifically designed for multimodal data integration.
- Algorithms: Advanced algorithms merge and process different modalities, using techniques like data fusion to create unified outputs.
Data Fusion Techniques
- Early Fusion: Combines raw data from multiple sources at the input stage (e.g., pairing an image with its caption).
- Mid Fusion: Fuses data during the learning phase after initial processing.
- Late Fusion: Processes each modality separately before merging outputs for final analysis.
Training Multimodal Models
These models are trained on vast datasets that cross-reference modalities (e.g., images paired with descriptive text or audio clips), allowing them to learn associations and context across different data types.
Watch this introductory video explaining multimodal AI concepts and demos:
Key Technologies Powering Multimodal AI
- Transformers: Neural network architectures that excel at processing sequential data and are now adapted for multimodal tasks.
- Convolutional Neural Networks (CNNs): Specialized for extracting features from images and video.
- Natural Language Processing (NLP): Enables understanding and generating human language.
- Shared Embedding Spaces: Allow different data types to be represented in a way that models can understand relationships between them.

Transformers are a core technology for multimodal AI.
Examples of Multimodal AI in Action
Model/Platform | Modalities Integrated | Key Use Case | Demo Video |
---|---|---|---|
OpenAI GPT-4o | Text, images, audio | Conversational AI, image captioning | Demo Video |
Google Gemini | Text, images, video | Search, content creation | Gemini Demo |
Meta ImageBind | Text, audio, images, video, sensors | Content creation, environmental sensing | — |
OpenAI CLIP | Images, text | Visual search, image-text alignment | — |
Runway Gen-2 | Text, video | Video generation from text prompts | — |
Real-World Applications
- Healthcare: Analyze medical images, patient records, and voice notes for more accurate diagnoses and personalized care.
- Retail: Enhance product recommendations by analyzing customer reviews, images, and browsing behavior.
- Customer Service: Provide richer, context-aware responses by combining chat transcripts, voice calls, and customer history.
- Entertainment: Generate videos from text prompts, create music from images, or design immersive AR/VR experiences.
- Autonomous Vehicles: Integrate sensor data, camera feeds, and map information for safer navigation.

Autonomous vehicles use multimodal data from cameras, lidar, radar, and more.
Watch an interview on advanced video understanding with multimodal AI:
Learn how to build interactive multimodal video chat systems in this course:
How Multimodal AI Differs from Traditional AI
Feature | Traditional (Unimodal) AI | Multimodal AI |
---|---|---|
Data Types | Single (text, image, or audio) | Multiple (text, image, audio, video, sensors) |
Context Awareness | Limited | Rich, human-like |
Output Accuracy | Lower | Higher, more nuanced |
Application Scope | Narrow | Broad, cross-domain |
Benefits of Multimodal AI
- Greater Contextual Understanding: By combining modalities, AI systems can interpret ambiguous or complex scenarios more effectively.
- Improved User Experience: Enables more natural and intuitive interactions with technology, such as voice assistants that understand images or chatbots that interpret tone and context.
- Versatility: Applicable across industries and adaptable to new data sources as technology evolves.
Challenges and Limitations
- Data Alignment: Ensuring that data from different modalities is properly synchronized and relevant to the same context can be complex.
- Computational Resources: Multimodal models require significant computing power and large datasets for effective training.
- Bias and Fairness: Integrating diverse data types can introduce new biases, making ethical considerations and robust evaluation essential.
- Interpretability: As models become more complex, understanding how decisions are made becomes more challenging.
The Future of Multimodal AI
- Smarter Assistants: Virtual agents capable of understanding and responding to text, voice, images, and even gestures in real time.
- Creative Tools: AI-powered platforms that generate art, music, and video content from simple prompts, revolutionizing creative industries.
- Personalized Experiences: Hyper-personalized healthcare, education, and entertainment tailored to individual needs and contexts.
- Safer Automation: Enhanced safety and efficiency in autonomous vehicles, robotics, and industrial automation through better situational awareness.
Explore advanced video AI applications and embedding APIs for deeper video understanding:
Twelve Labs: Mastering Multimodal AI for Advanced Video – Databricks Blog
Conclusion
Multimodal AI represents a significant leap forward in artificial intelligence, bridging the gap between how machines and humans perceive the world. By integrating multiple data types, these systems deliver richer insights, more accurate predictions, and transformative user experiences. As the technology matures, its impact will be felt across every sector, driving innovation and shaping the future of human-machine interaction.
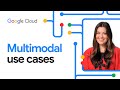
No comments: