Why Multimodal AI Is Smarter Than Any Tech Before Unlock the Future: Why Multimodal AI Is Outperforming Every Tech Ever Created!
Why Multimodal AI Is Smarter Than Any Tech Before
Exploring how multimodal AI integrates diverse data to outperform previous technologies
What Is Multimodal AI?
Multimodal AI refers to systems that process and integrate data from multiple sources or “modalities.” These can include:
- Text (documents, chat, code)
- Images (photos, diagrams)
- Audio (speech, music, environmental sounds)
- Video (combining visual and audio streams)
- Sensor data (from IoT devices, wearables, etc.)
Unlike unimodal AI, which is limited to a single data type, multimodal AI can combine these inputs for a richer, more accurate understanding of context and meaning.
How Multimodal AI Works
Multimodal AI uses advanced machine learning techniques to fuse information from different sources. For example, it might:
- Analyze an image and its caption together for better scene understanding.
- Combine audio and video to improve speech recognition in noisy environments.
- Integrate sensor data with visual feeds for robotics and autonomous vehicles.
Core techniques include:
- Self-attention and transformers for cross-modal learning.
- Variational autoencoders (VAEs) for generating new content across modalities.
- Graph neural networks (GNNs) for modeling relationships between diverse data types.
Google Gemini: Multimodal Reasoning Demo
Source: BackstageTV (Mar 2025)
Key Architectures and Models
Some of the most advanced multimodal AI systems include:
- Google DeepMind Gemini: Designed from scratch for seamless reasoning across text, images, video, audio, and code. Gemini Ultra outperformed human experts on the MMLU benchmark, showcasing its human-level multitasking abilities.
- Meta’s ImageBind: Processes six modalities (text, image, video, thermal, depth, audio) for comprehensive understanding.
- OpenAI’s CLIP: Aligns text and image embeddings, enabling zero-shot image classification and text-to-image tasks.
- Flamingo (DeepMind): Excels at visual question answering by integrating text and images.
- DALL·E: Generates images from textual prompts, demonstrating deep cross-modal creativity.
Meta ImageBind: 6-Modality Integration
Source: HeIIo (Dec 2023)
Real-World Applications
Healthcare
AI models like Med-PaLM 2 combine patient records, imaging (X-rays, CT), and genomic data for accurate diagnostics.
Autonomous Vehicles
Tesla and Waymo use multimodal AI, fusing data from cameras, radar, LiDAR, and audio sensors for real-time navigation.
Business & Productivity
Microsoft Copilot and Salesforce Einstein integrate voice, text, and visual data for smarter document creation and customer analytics.
Creative Industries
Text-to-image and text-to-video models (like DALL·E and Sora) enable designers to generate visuals from simple prompts.
Accessibility
Image captioning and speech-to-text tools help people with disabilities interact with digital content more easily.
Gemini in Creative Problem-Solving
Source: BackstageTV (Mar 2025)
Why Multimodal AI Outperforms Previous Tech
Feature | Unimodal AI | Multimodal AI |
---|---|---|
Input Types | Single (e.g., text only) | Multiple (text, image, audio) |
Context Understanding | Limited | Deep, human-like |
Robustness | Lower (single point of failure) | Higher (redundancy across modalities) |
Creativity | Restricted | Cross-modal generation |
Real-World Adaptability | Narrow | Broad, dynamic |
Key advantages:
- Contextual Intelligence: Multimodal AI resolves ambiguities by referencing multiple data streams, just as humans do.
- Robustness: If one input is missing or noisy, others can compensate, making systems more reliable.
- Personalization: AI can adapt to user preferences by analyzing speech, facial expressions, and written feedback together.
- Generative Power: Text-to-image, text-to-audio, and text-to-video generation are only possible with multimodal understanding.
Challenges and Future Trends
Challenges
- Data alignment and synchronization across modalities.
- High computational and data requirements.
- Bias and fairness in training data.
Future Trends
- Real-time multimodal applications (e.g., live translation, AR/VR).
- Greater integration with IoT and edge devices.
- More open-source and accessible multimodal models.
Essential Tools & Frameworks

TensorFlow Extended (TFX)
End-to-end platform for deploying multimodal pipelines.
Hugging Face Transformers
State-of-the-art multimodal models and APIs.
PyTorch Lightning
Scalable training for multimodal AI systems.
Videos & Further Reading
Images & Visual Examples

Source: Medium – Multimodal AI Architecture

Source: Medium – Unimodal vs Multimodal AI

Source: DeepMind – Google Gemini
Conclusion
“Multimodal AI is not just an upgrade—it’s a transformation. By merging multiple senses into one intelligent system, it delivers smarter decisions, more natural interactions, and unprecedented robustness.”
Multimodal AI marks a new era in artificial intelligence, enabling systems to understand, reason, and create across multiple data types with human-like intelligence. Its ability to integrate and interpret diverse information streams makes it vastly superior to previous single-modality technologies, unlocking new possibilities in healthcare, business, creativity, and beyond.
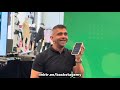
No comments: